Podium finish in global AI race contest
Story by Kurtis Eichler and Eddie Major
An AIML team has scored double pole positions in a global virtual motor racing event that saw hundreds of AI researchers and engineers compete to build high performance virtual race cars.
The team, made up of University of Adelaide postgraduate research students and AIML machine learning engineers, beat more than 400 international competitors to secure first and second places in both race categories in the final stage of the Learn-to-Race Autonomous Racing Virtual Challenge in April.
The challenge saw teams build virtual agents that learned how to drive on a computer-generated racetrack using vehicle speed and images of the track from a driver’s perspective.
Cars then competed across new and previously unseen digital racing environments modelled on real-world race tracks, such as the Las Vegas Motor Speedway and Thruxton Circuit in the UK.
University of Adelaide postgraduate students Matthew Howe, Adrian Orenstein and James Bockman, and AIML machine learning engineers Lachlan Mares, Stefan Podgorski, and Sam Bahrami, worked on the challenge in their spare time because they wanted to build and test their AI skills in a global open challenge environment.
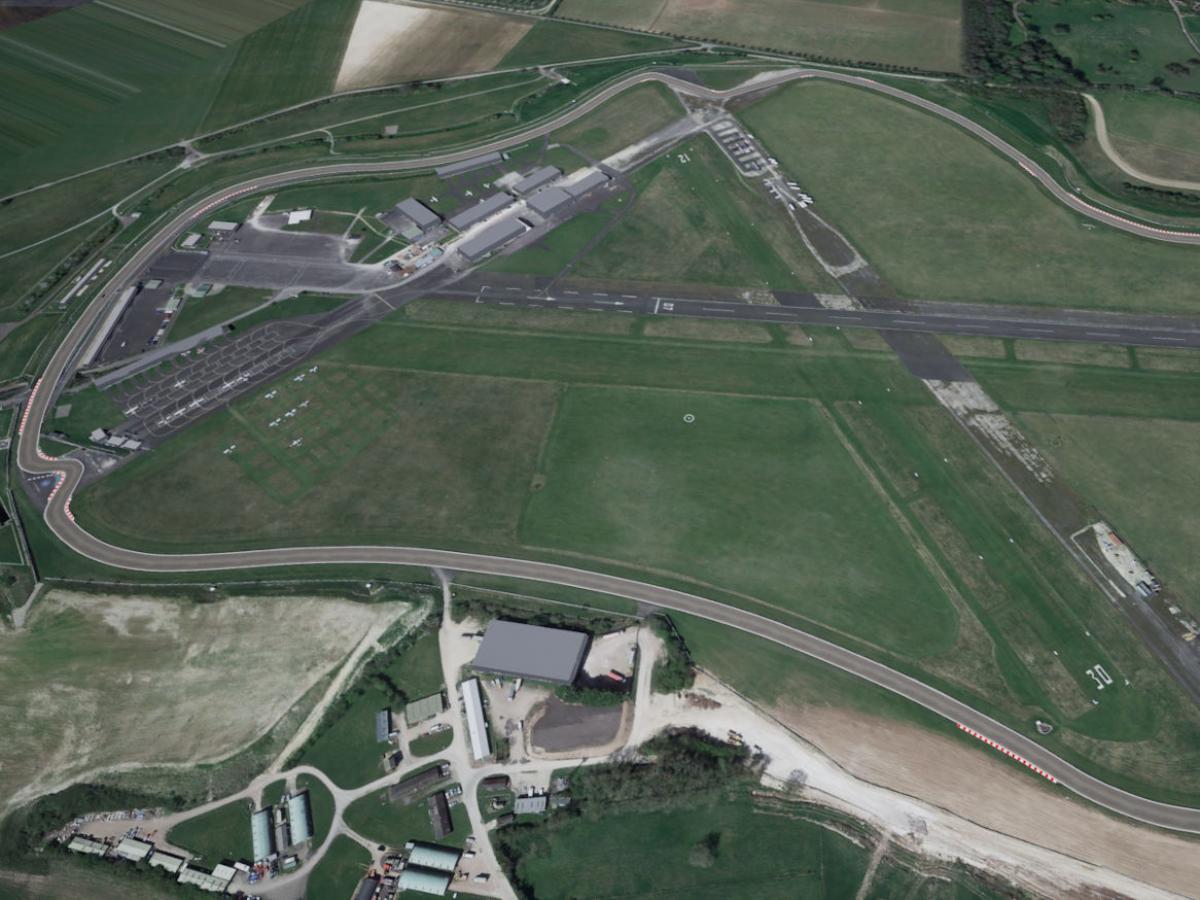
The teams built virtual agents that learned how to drive on a computer-generated racetrack based on Thruxton Circuit in the UK.
“Autonomous racing has always been a goal of ours,” Howe says.
“I’ve also wanted to be involved in a roborace for a while as well, so when this challenge came up, it was pretty exciting.”
The challenge required the team to build something called a reinforcement learning agent — a type of machine learning that rewards the agent’s correct behaviour, and punishes any undesired behaviour. Effective reinforcement learning agents are able to perceive and interpret their environment (in this case, the race track) from input data and learn through a process of trial and error.
The event organisers say the objective of the challenge is to “push the boundary of autonomous technology”, with a focus on “achieving the safety benefits of autonomous driving”.
Howe says the team spent five months working on their solution.
“It was very satisfying for it to be finished and for us to place pretty highly,” he says.
“We’re all students, and there’s not a group of people at AIML that particularly work on autonomous racing.
This challenge came just months after Howe teamed up with Orenstein and Bockman for the Amazon Web Services DeepRacer contest, a week-long autonomous racing challenge.
“(That challenge) was very restrictive and was very reinforcement learning-focused and we kind of only had this very poor simulation of vehicle dynamics that we had access to,” Bockman says.
“So, this was kind of the natural extension and more of a real simulation with proper aerodynamics on the car and proper tyre models.”
For research engineer Lachlan Mares the challenge was an opportunity to build and test his software engineering skills at an international competition level.
“I guess the bigger thing was the learning that I took away from these guys, who are very good machine learning researchers. I just did it for the experience,” Mares says.
AIML’s director, Professor Simon Lucey, is also a principal scientist at Argo AI—the multi billion dollar Pittsburgh-based autonomous driving company backed by Ford and Volkswagen—and says AI competitions are important for both research and the tech industry.
“We know our research is extremely competitive at an international level, but ranked challenges are a great way to test and demonstrate applied skills in building software solutions for real-world projects,” Professor Lucey says.
He says competitions are also of interest to tech companies seeking to recruit the best machine learning researchers and engineers.
“It shows the world where the best talent is, and often, that’s here in South Australia.”
Autonomous driving technology is growing in demand across automotive and transport industries, and the point of this challenge is to test safety, performance and capabilities of reinforced learning agents.
“These challenges are great for bringing together all of those ideas and expertise from all the different areas and trying to build an entire solution,” Howe says.
The Learn-to-Race Challenge is organised and hosted by data science competition platform AICrowd, and sponsored by Carnegie Mellon University, Arrival and Amazon Web Services.
AIML and University of Adelaide research and engineering teams regularly place highly in international machine learning challenges. Examples include the European Space Agency Satellite Pose Estimation Challenge, the NASA Space Robotics Challenge, REFUGE Retinal Fundus Glaucoma Challenge, and VQA 2.0 Challenge.