Algorithmic audit for safer medical AI systems
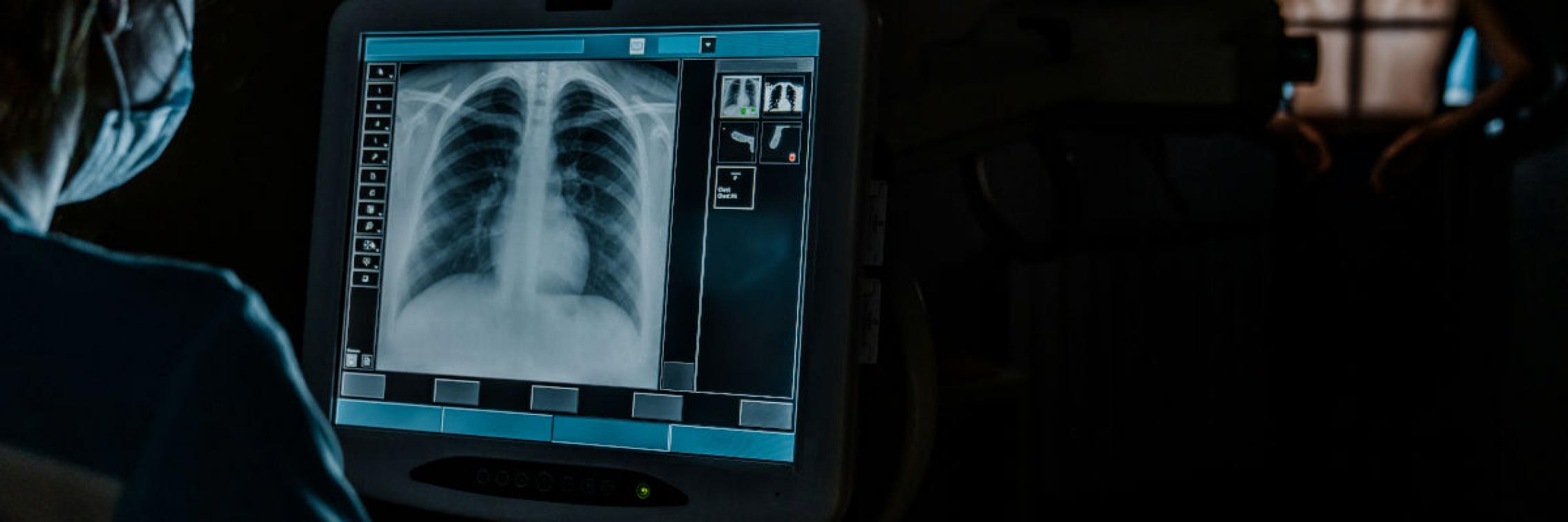
An artificial intelligence (AI) model trained to detect hip fractures from x-rays can outperform highly trained clinical specialists, but is also vulnerable to unexpected and potentially harmful errors, highlighting the need for ‘algorithmic audits’ of medical AI imaging systems, researchers say.
Dr Lauren Oakden-Rayner, a practising clinical radiologist and Senior Research Fellow in medical AI with the University of Adelaide’s Australian Institute for Machine Learning (AIML), is a lead author of two papers published last month in Lancet Digital Health.
Medical AI refers to the development of mathematical algorithms and models that can interpret medical data, such as x-rays, for the purpose of improving diagnosis and patient outcomes.
In one paper, Dr Oakden-Rayner worked with a team of researchers—including AIML’s Director of Medical Machine Learning Professor Gustavo Carneiro, and University of Adelaide Professor of Genetic Epidemiology Lyle Palmer—to conduct a diagnostic accuracy study of a custom AI model trained to detect hip fractures from x-rays.
Hip fractures are a significant public health burden, and a frequent cause of hospitalisation for older people, carrying a lifetime risk of 17.5% for women and 6% for men. However, one in 10 suspected hip fractures are not diagnosed from the initial pelvic x-ray, requiring the patient to undergo further imaging.
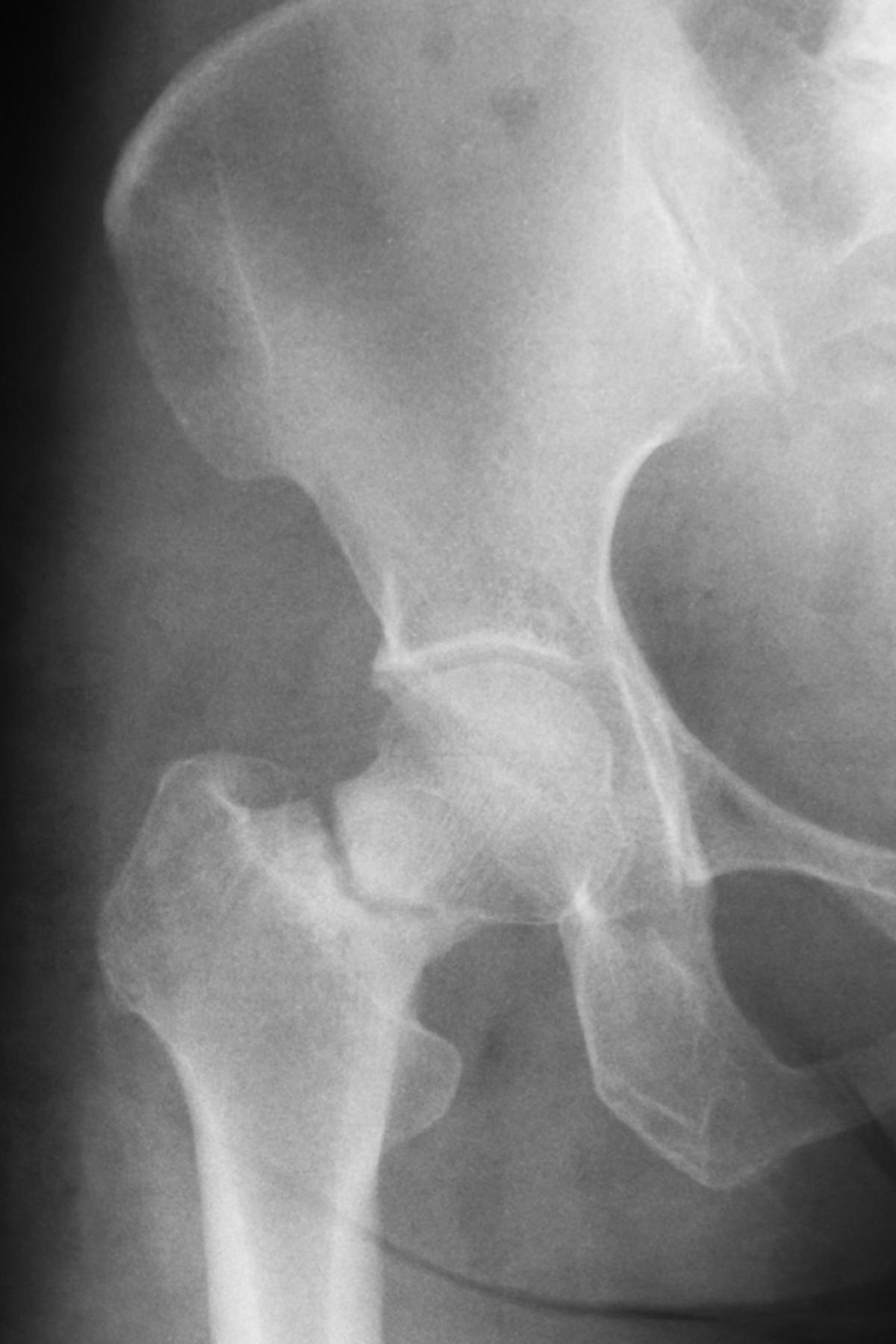
An X-ray image showing a femoral neck fracture, or broken hip. This is a stock photograph that was not used in the research study. Photo: iStock
Known as a deep learning model (a type of AI that can learn to perform classification tasks from images, video and sound) the system was trained using a dataset of more than 45,000 hip x-rays from the emergency department of the Royal Adelaide Hospital.
Thirteen experienced doctors also reviewed a smaller number of x-rays under similar conditions to their normal clinical practice. The AI system outperformed the human doctors, and was able to correctly identify 95.5% of hip fractures (sensitivity) compared to 94.5% for the best radiologist; and correctly identified 99.5% of x-rays with non-broken bones (specificity) compared to 97% for the doctors.
However, the study also revealed concerning model failure modes—circumstances in which an AI system fails repeatably under specific conditions—where the deep learning model wasn’t able to diagnose obviously broken bones, and misdiagnosed patients with unrelated bone disease.
“The high-performance hip fracture model fails unexpectedly on an extremely obvious fracture and produces a cluster of errors in cases with abnormal bones, such as Paget’s disease,” Dr Oakden-Rayner said.
“These findings, and risks, were only detected via audit.”
Almost 200 medical AI products are currently FDA-cleared for use in medical imaging in the U.S., including systems for identifying bone fractures, measuring heart blood flow, surgical planning, and diagnosing strokes. The risk highlighted in this study, that high performance AI systems can yield unexpected errors that might be missed without proactive and robust investigation and auditing, is not currently addressed in existing laws and regulations.
In another paper also published in Lancet Digital Health, Dr Oakden-Rayner and her colleagues propose a medical algorithmic audit framework to guide users, developers, and regulators through the process of considering potential errors in medical diagnostic systems, mapping what components may contribute to the errors, and anticipating potential consequences for patients.
Dr Oakden-Rayner says that algorithmic audit research is already informing industry standards for safely using AI systems in health care.
“We’re excited that this work is impacting policy. Professional organisations such as the Royal Australian and New Zealand College of Radiologists are incorporating audit into their practice standards, and we’re talking with regulators and governance groups on how audit can make AI systems safer,” Dr Oakden-Rayner said.
The authors propose that safety monitoring and auditing should be a joint responsibility between users and developers, and that this should be “part of a larger oversight framework of algorithmovigilance to ensure the continued efficacy and safety of artificial intelligence systems.”
—
Oakden-Rayner, L., Gale, W., Bonham, T., Lungren, M., Carneiro, G., Bradley, A. and Palmer, L., 2022. Validation and algorithmic audit of a deep learning system for the detection of proximal femoral fractures in patients in the emergency department: a diagnostic accuracy study. The Lancet Digital Health, 4(5), pp.e351-e358.
Liu, X., Glocker, B., McCradden, M., Ghassemi, M., Denniston, A. and Oakden-Rayner, L., 2022. The medical algorithmic audit. The Lancet Digital Health, 4(5), pp.e384-e397.