From avocado armchairs to Studio Ghibli dreams
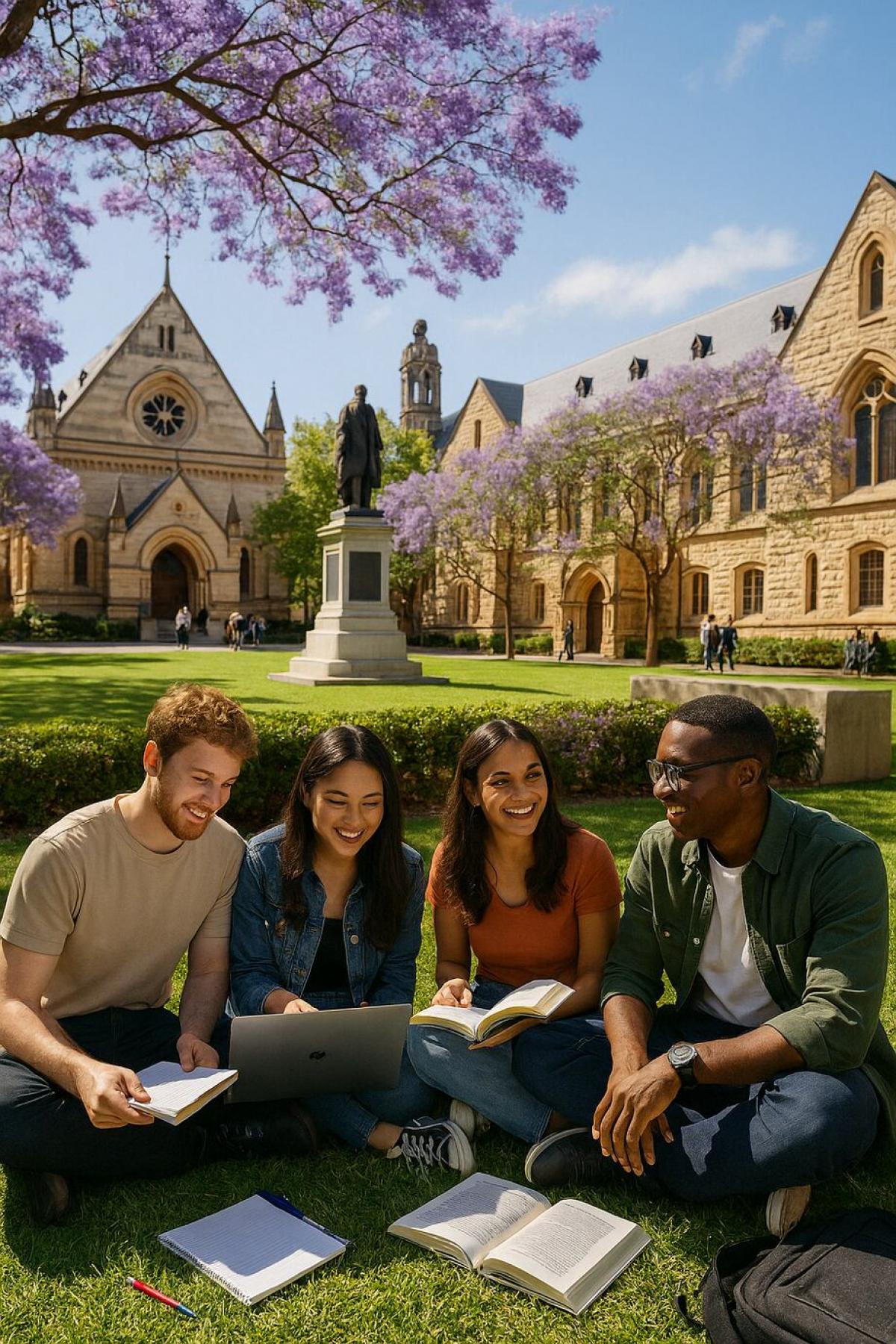
What even is a photograph anymore? At first glance it looks like a beautiful summer's day on campus. But these students don't actually exist, as this synthetic photo was produced in just minutes using ChatGPT's new image generation feature.
AI image generation capability just leapt ahead, what will it mean for teaching?
Earlier this month the internet was suddenly flooded with a new kind of portrait: Japanese anime-style illustrations of tech founders, celebrities, influencers, re-imagined memes, and even a president or two.
The reason? OpenAI had delivered a major update to the image generation capabilities of ChatGPT, and users discovered they could now generate very high-quality, richly styled images—including ‘Ghiblified’ versions of themselves—with just a simple written prompt. It was a breakthrough in AI image generation capability, and one which will impact some of the fundamental ways we deal with visual information which will take some time to unpack.
But first, how did we get here? It’s not the beginning, but let’s start with an avocado armchair.
Back in 2021, well before ChatGPT, AI tech startup OpenAI released an AI tool called DALL.E. It was built by training it on a massive dataset of paired text and image captions, allowing it to learn the relationship between language and visual elements. While trying to understand and test the limits of their new tool's abilities, an OpenAI researcher started asking it to generate images it wouldn’t have seen in its training data, such as an armchair that looks like an avocado.
The results might look a little clunky to us today, but DALL.E was able to combine two discrete and unrelated elements into geometrically plausible novel images; a stunning result. The avocado armchair became a mascot for AI image generation capability, and helped launch the idea of text-to-image generation into public awareness.
While there have been multiple advancements in AI image generators since then—Midjourney and Stable Diffusion in mid-2022 are notable—ChatGPT’s new 4o Image Generation model stands out as a significant milestone. It can generate stunningly photorealistic images or accurately imitate countless visual styles from throughout art history, all while following complex prompts with surprising accuracy.

Images produced by OpenAI's DALL.E software in 2021, demonstrating how the AI model could successfully combine unrelated concepts—in this case, avocados and armchairs—into coherent novel outputs. DALL.E was one of the first examples of an AI model showing early-stage generalisation across unseen concept pairings at scale.
Understanding the impact of multimodal AI
When you experiment with ChatGPT’s 4o Image Generation for the first time, you’re impressed (stunned?) by the results. But things really get exciting when you realise it’s a two-way street. It doesn’t just create images upon request (text-to-image), it’s multimodal.
The unified AI model architecture can process and respond to different types of input simultaneously—text, images and audio—allowing it to not only generate pictures from a written description but also ‘understand’ uploaded photos (and their geometric relationships in a scene); answer questions about diagrams, maps and graphs; and accurately translate between languages (oral and written).
Ok. So, what’s the bad news?
Is everyone enjoying this new technology? Not even close! There are many detractors who are concerned about what’s happening in AI tech and voicing their criticisms loudly (Hayao Miyazaki, the respected animator and founder of Studio Ghibli, described AI in animation as "utterly disgusting" and "an insult to life itself").
A short list of just some of the problematic issues might include:
- Legal - AI tech companies claim their use of hundreds of millions of books, photographs, movies, artworks and other visual property to train their AI models is permissible under existing copyright legislation. Content creators feel differently, and the lawsuits are numerous.
- Workforce and welfare - Even if legal under intellectual property law, multimodal AI is likely to displace whole creative industries, affecting the welfare of actors, artists, musicians, photographers, graphic designers and artists.
- Moral and ethical - People and communities aren’t able to decide if their visual culture gets ‘used’ by AI technology, that’s led to allegations of digital colonialism and cultural appropriation.
- Environmental - Multimodal AI systems use a lot of energy during training and operation, and that has people concerned about the environmental impact of their AI use.
- Disinformation and trust - Hyper-realistic AI-generated content can be used to fabricate news, create fake evidence, or impersonate individuals. This raises major concerns for academic integrity, media trust, and knowing how to tell what’s ‘real’ anymore.
- Bias and stereotyping - Because multimodal AI is trained on vast collections of existing artworks and photographs, they may reproduce biases present in those datasets and tend to reflect dominant cultural norms, stereotypes, or exclusions embedded in our visual history.
What does multimodal AI mean for university teaching?
Decades of research in cognitive psychology has shown that visual elements, when correctly applied, can improve understanding and memory when combined with text or spoken information. Exactly how the latest in AI image generation will be applied to augment learning activities will be interesting to see in the coming months, and no doubt we can expect another wave of research publications on the horizon soon (see some links to preprints below).
But even educators who don’t actively facilitate learning with visuals will benefit from building an understanding of how multimodal AI is going to have some impact across all disciples.
Our AI Literacy Framework (AILF) offers one way to approach what this might mean for students. The following dot points are potential multimodal AI competencies aligned to the AILF’s four dimensions:
Recognise and Understand
- Recognise that modern AI-generated images are highly realistic and can no longer be detected through visual inspection alone; it requires contextual awareness and critical questioning.
Use and Apply
- Learn to prompt image models with specificity and iterate to align outputs with context and purpose—just as they might with text-based tools.
- Apply generative images ethically and transparently, acknowledging the role of AI in their creation and ensuring visual content is appropriate and accurate.
Evaluate and Critique
- Question the authenticity and accuracy of visual media, especially in academic or professional contexts.
- Understand how AI-generated visuals may be used to persuade or mislead, especially in the context of social media, politics, or advertising.
Reflect and Respect
- Reflect on how synthetic images could be used to exploit, manipulate, or erase marginalised identities, and how to guard against that.
- Recognise the ethical issues and cultural appropriation may arise when mimicking artistic styles or producing images of real individuals, and what this may mean for specific communities.
- Consider the environmental impact of generating images at scale, especially with large, compute-intensive models.
Further reading
Chan, J. & Li, Y. (2025) Enhancing Higher Education with Generative AI: A Multimodal Approach for Personalised Learning. arXiv preprint.
Chen, S. et al. (2025) An empirical study of GPT-4o image generation capabilities. arXiv preprint.
OpenAI. (2025). Addendum to GPT-4o System Card: 4o image generation.
Lee, G. et al. (2023) Multimodality of AI for Education: Towards Artificial General Intelligence. arXiv preprint.
We’re sharing these articles to profile the different ways educators are approaching generative AI in their teaching practice. Before using any gen AI software tools, University of Adelaide staff should understand the ITDS Generative AI IT Security Guidelines and ensure they maintain information security and data privacy.
If you’re encouraging students to use gen AI tools in their studies, be mindful of how varying levels of access to software (including paid subscriptions) might impact education equity among diverse student cohorts.
Feel free to encourage your students to check out the University Library’s Guide for using AI for study and research in an ethical, responsible and evaluative way.
Further support materials can be found on the AI and Learning website (staff login required).